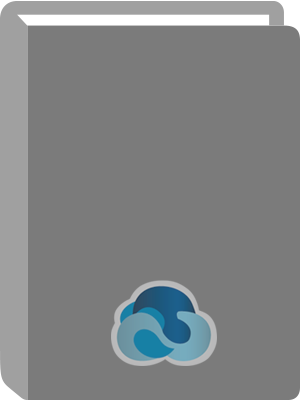
Başlık:
New directions in spatial econometrics
Yazar:
Anselin, Luc, 1953-, editor.
ISBN:
9783642798795
Fiziksel Tanımlama:
xix, 420 pages. : illustration., maps ; 24 cm.
Seri:
Advances in spatial science.
İçerik:
1 New Directions in Spatial Econometrics: Introduction.- 1.1 Introduction.- 1.2 Spatial Effects in Regression Models.- 1.2.1 Specification of Spatial Dependence.- 1.2.2 Spatial Data and Model Transformations.- 1.3 Spatial Effects in Limited Dependent Variable Models.- 1.4 Heterogeneity and Dependence in Space-Time Models.- 1.5 Future Directions.- References.- I-A: Spatial Effects in Linear Regression Models Specification of Spatial Dependence.- 2 Small Sample Properties of Tests for Spatial Dependence in Regression Models: Some Further Results.- 2.1 Introduction.- 2.2 Tests for Spatial Dependence.- 2.2.1 Null and Alternative Hypotheses.- 2.2.2 Tests for Spatial Error Dependence.- 2.2.3 Tests for Spatial Lag Dependence.- 2.3 Experimental Design.- 2.4 Results of Monte Carlo Experiments.- 2.4.1 Empirical Size of the Tests.- 2.4.2 Power of Tests Against First Order Spatial Error Dependence.- 2.4.3 Power of Tests Against Spatial Autoregressive Lag Dependence.- 2.4.4 Power of Tests Against Second Order Spatial Error Dependence.- 2.4.5 Power of Tests Against a SARMA (1,1) Process.- 2.5 Conclusions.- Acknowledgements.- References.- Appendix 1: Tables.- 3 Spatial Correlation: A Suggested Alternative to the Autoregressive Model.- 3.1 Introduction.- 3.2 The Spatial AR Model of Autocorrelation.- 3.3 The Singularity of (I - pM).- 3.3.1 Theoretical Issues.- 3.3.2 Independent Corroborative Evidence.- 3.4 The Parameter Space.- 3.5 A Suggested Variation of the Spatial AR Model.- 3.5.1 The Suggested Model.- 3.5.2 Some Limiting Correlations.- 3.5.3 A Generalization.- 3.6 Suggestions for Further Work.- Acknowledgements.- References.- Appendix 1: Spatial Weighting Matrices.- 4 Spatial Autoregressive Error Components in Travel Flow Models: An Application to Aggregate Mode Choice.- 4.1 Introduction.- 4.2 The First-Order Spatially Autoregressive Error Components Formulation.- 4.3 Estimation Issues.- 4.4 Empirical Example.- 4.4.1 An Illustration Based on Synthetic Data.- 4.5 Conclusions.- References.- I-B: Spatial Effects in Linear Regression Models Spatial Data and Model Transformations.- 5 The Impacts of Misspecified Spatial Interaction in Linear Regression Models.- 5.1 Introduction.- 5.2 Aggregation and the Identification of Spatial Interaction.- 5.3 Experimental Design.- 5.3.1 Sample Size.- 5.3.2 Spatial Interaction Structures.- 5.3.3 Spatial Models and Parameter Space.- 5.3.4 Test Statistics and Estimators.- 5.3.5 Forms of Misspecification.- 5.4 Empirical Results.- 5.4.1 Size of Tests Under the Null.- 5.4.2 Power of Tests.- 5.4.3 Misspecification Effects on the Power of Tests for Spatial Dependence.- 5.4.4 Sensitivity of Parameter Estimation to Specification of Weight Matrix.- 5.4.5 Impact of Misspecification of Weight Matrix on Estimation.- 5.5 General Inferences References.- 6 Computation of Box-Cox Transform Parameters: A New Method and its Application to Spatial Econometrics.- 6.1 Introduction.- 6.2 The Elasticity Method: Further Elaboration.- 6.2.1 Linearization Bias.- 6.2.2 Discretization Bias.- 6.2.3 Specification Bias.- 6.3 The One Exogenous Variable Test.- 6.4 An Application to Spatial Econometrics.- 6.5 The Multiple Exogenous Variable Computation.- 6.6 Conclusions.- References.- 7 Data Problems in Spatial Econometric Modeling.- 7.1 Introduction.- 7.2 Data for Spatial Econometric Analysis.- 7.3 Data Problems in Spatial Econometrics.- 7.4 Methodologies for Handling Data Problems.- 7.4.1 Influential Cases in the Standard Regression Model.- 7.4.2 Influential Cases in a Spatial Regression Model.- 7.4.3 An Example.- 7.5 Implementing Methodologies.- References.- 8 Spatial Filtering in a Regression Framework: Examples Using Data on Urban Crime, Regional Inequality, and Government Expenditures.- 8.1 Introduction.- 8.2 Rationale for a Spatial Filter.- 8.3 The Gi Statistic.- 8.4 The Filtering Procedure.- 8.5 Filtering Variables: Three Examples.- 8.5.1 Example 1: Urban Crime.- 8.5.2 Example 2: Regional Inequality.- 8.5.3 Example 3: Government Expenditures.- >8.6 Conclusions.- >Acknowledgments.- References.- II: Spatial Effects in Limited Dependent Variable Models.- 9 Spatial Effects in Probit Models: A Monte Carlo Investigation.- 9.1 Introduction.- 9.2 Sources of Heteroscedasticity.- 9.3 Heteroscedastic Probit.- 9.4 Monte Carlo Design.- 9.5 Tests.- 9.6 Monte Carlo Results.- 9.7 Conclusions.- References.- Appendix 1: Monte Carlo Results.- Appendix 2: Heteroscedastic Probit Computer Programs.- Appendix 3: Monte Carlo Computer Programs.- 10 Estimating Logit Models with Spatial Dependence.- 10.1 Introduction.- 10.1.1 Model.- 10.2 Simulation Example.- 10.3 Conclusions.- >References.- Appendix 1: Gauss Program for Finding ML Estimates.- Appendix 2: Gauss Program to Estimate Asymptotic Variances of ML Estimates.- 11 Utility Variability within Aggregate Spatial Units and its Relevance to Discrete Models of Destination Choice.- 11.1 Introduction.- 11.2 Theoretical Background.- 11.3 Estimation of the Maximum Utility Model.- 11.4 Model Specifications and Simulations.- 11.4.1 Specification Issues.- 11.4.2 Description of Simulation Method.- 11.4.3 Results.- 11.5 Conclusions.- Acknowledgement.- References.- III: Heterogeneity and Dependence in Space-Time Models.- 12 The General Linear Model and Spatial Autoregressive Models.- 12.1 Introduction.- 12.2 The GLM.- 12.3 Data Preprocessing.- 12.3.1 Analysis of the 1964 Benchmark Data.- 12.3.2 Evaluation of Missing USDA Values Estimation.- >12.4 Implementation of the Spatial Statistical GLM.- 12.4.1 Preliminary Spatial Analysis of Milk Yields: AR Trend Surface GLMs.- 12.4.2 AR GLM Models for the Repeated Measures Case.- 12.4.3 A Spatially Adjusted Canonical Correlation Analysis of the Milk Production Data.- 12.5 Conclusions.- >References.- >Appendix 1: SAS Computer Code to Compute the Popular Spatial Autocorrelation Indices.- Appendix 2: SAS Code for Estimating Missing Values in the 1969 Data Set.- Appendix 3: SAS Code for 1969 USDA Data Analysis.- 13 Econometric Models and Spatial Parametric Instability: Relevant Concepts and an Instability Index.- 13.1 Introduction.- 13.2 The Expansion Method.- 13.3 Parametric Instability.- 13.3.1 Example.- 13.4 Conclusions.- 13.4.1 Instability Measures: Scope.- 13.4.2 Instability Measures: Significance.- References.- 14 Bayesian Hierarchical Forecasts for Dynamic Systems: Case Study on Backcasting School District Income Tax Revenues.- 14.1 Introduction.- 14.2 Literature Review.- 14.3 The C-MSKF Model: Time Series Prediction with Spatial Adjustments.- 14.3.1 Multi-State Kaiman Filter.- 14.3.2 Spatial Adjustment via Hierarchical Random Effects Model.- 14.3.3 CIHM Method.- 14.3.4 C-MSKF.- 14.4 Case Study and Observational Setting.- 14.4.1 Data.- 14.4.2 Treatments.- 14.5 Results.- >14.6 Conclusions.- >References.- Appendix 1: Poolbayes Program.- 15 A Multiprocess Mixture Model to Estimate Space-Time Dimensions of Weekly Pricing of Certificates of Deposit.- 15.1 Introduction.- 15.2 A Dynamic Targeting Model of CD Rate-Setting Behavior.- 15.2.1 The Model.- 15.2.2 The Decision Rule.- 15.3 The Spatial Econometric Model.- 15.3.1 Spatial Time-Varying Parameters.- 15.3.2 Parameter Estimation.- 15.3.3 Testing Hypotheses with the Model.- 15.4 Implementing the Model.- 15.4.1 The Data.- 15.4.2 Prior Information.- 15.4.3 Empirical Results.- 15.5 Conclusions.- Acknowledgements.- References.- Appendix 1: FORTRAN Program for the Spatial Mixture.- Author Index.- Contributors.
Özet:
The promising new directions for research and applications described here include alternative model specifications, estimators and tests for regression models and new perspectives on dealing with spatial effects in models with limited dependent variables and space-time data.
Copies:
Mevcut:*
Library | Materyal Türü | Demirbaş | Yer Numarası | Durumu / Lokasyon / İade Tarihi |
---|---|---|---|---|
Arıyor... | Kitap | EKOBKN0007453 | 330.015195 NEW 1995 | Arıyor... |