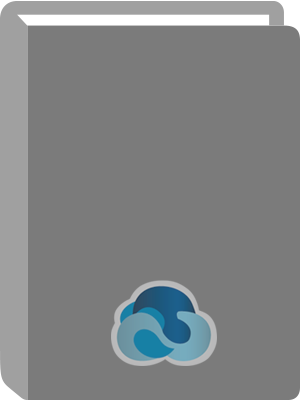
Başlık:
Age-period-cohort analysis : new models, methods, and empirical applications
Yazar:
Yang, Yang, 1975- author
ISBN:
9781466507524
Yazar Ek Girişi:
Fiziksel Tanımlama:
xiii, 338 pages : illustrations, charts ; 24 cm
İçerik:
1 Introduction 1 (6) References 5 (2) 2 Why Cohort Analysis? 7 (8) 2.1 Introduction 7 (1) 2.2 The Conceptualization of Cohort Effects 7 (2) 2.3 Distinguishing Age, Period, and Cohort 9 (3) 2.4 Summary 12 (1) References 13 (2) 3 APC Analysis of Data from Three Common Research Designs 15 (40) 3.1 Introduction 15 (1) 3.2 Repeated Cross-Sectional Data Designs 15 (4) 3.3 Research Design I: Age-by-Time Period Tabular Array of Rates/Proportions 19 (7) 3.3.1 Understanding Cancer Incidence and Mortality Using APC Analysis: Biodemography, Social Disparities, and Forecasting 19 (2) 3.3.2 Cancer Incidence Rates from Surveillance, Epidemiology, and End Results (SEER): 1973-2008 21 (1) 3.3.3 Cancer Mortality Rates from the National Center for Health Statistics (NCHS): 1969-2007 21 (5) 3.4 Research Design II: Repeated Cross-Sectional Sample Surveys 26 (13) 3.4.1 General Social Survey (GSS) 1972-2006: Verbal Test Score and Subjective Well-Being 26 (6) 3.4.2 National Health and Nutrition Examination Surveys (NHANES) 1971-2008: The Obesity Epidemic 32 (2) 3.4.3 National Health Interview Surveys (NHIS) 1984-2007: Health Disparities 34 (3) 3.4.4 Birth Cohort and Time Period Covariates Related to Cancer Trends 37 (2) 3.5 Research Design III: Prospective Cohort Panels and the Accelerated Longitudinal Design 39 (11) 3.5.1 Americans' Changing Lives (ACL) Study 1986-2002: Depression, Physical Disability, and Self-Rated Health 41 (7) 3.5.2 Health and Retirement Survey (HRS) 1992-2008: Frailty Index 48 (2) References 50 (5) 4 Formalities of the Age-Period-Cohort Analysis Conundrum and a Generalized Linear Mixed Models (GLMM) Framework 55 (20) 4.1 Introduction 55 (1) 4.2 Descriptive APC Analysis 56 (5) 4.3 Algebra of the APC Model Identification Problem 61 (2) 4.4 Conventional Approaches to the APC Identification Problem 63 (5) 4.4.1 Reduced Two-Factor Models 64 (1) 4.4.2 Constrained Generalized Linear Models (CGLIMs) 65 (1) 4.4.3 Nonlinear Parametric Transformation 66 (1) 4.4.4 Proxy Variables 66 (1) 4.4.5 Other Approaches in Biostatistics 67 (1) 4.5 Generalized Linear Mixed Models (GLMM) Framework 68 (3) References 71 (4) 5 APC Accounting/Multiple Classification Model, Part I: Model Identification and Estimation Using the Intrinsic Estimator 75 (50) 5.1 Introduction 75 (1) 5.2 Algebraic, Geometric, and Verbal Definitions of the Intrinsic Estimator 76 (8) 5.2.1 Algebraic Definition 77 (3) 5.2.2 Geometric Representation 80 (2) 5.2.3 Verbal Description 82 (1) 5.2.4 Computational Tools 83 (1) 5.3 Statistical Properties 84 (5) 5.3.1 Estimability, Unbiasedness, and Relative Efficiency 84 (2) 5.3.2 Asymptotic Properties 86 (1) 5.3.3 Implications 87 (2) 5.4 Model Validation: Empirical Example 89 (3) 5.5 Model Validation: Monte Carlo Simulation Analyses 92 (17) 5.5.1 Results for APC Models: True Effects of A, P, and C All Present 94 (10) 5.5.1.1 Property of Estimable Constraints 98 (6) 5.5.2 Misuse of APC Models: Revisiting a Numerical Example 104 (5) 5.6 Interpretation and Use of the Intrinsic Estimator 109 (6) Appendix 5.1 Proof of Unbiasedness of the IE as an Estimator of the b0 = Pprojb Constrained APC Coefficient Vector 115 (1) Appendix 5.2 Proof of Relative Efficiency of the IE as an Estimator of the b0 = Pprojb Constrained APC Coefficient Vector 116 (1) Appendix 5.3 IE as a Minimum Norm Quadratic Unbiased Estimator of the b0 = Pprojb Constrained APC Coefficient Vector 117 (1) Appendix 5.4 Interpreting the Intrinsic Estimator, Its Relationship to Other Constrained Estimators in APC Accounting Models, and Limits on Its Empirical Applicability 118 (2) References 120 (5) 6 APC Accounting/Multiple Classification Model, Part II: Empirical Applications 125 (66) 6.1 Introduction 125 (1) 6.2 Recent U.S. Cancer Incidence and Mortality Trends by Sex and Race: A Three-Step Procedure 125 (44) 6.2.1 Step 1: Descriptive Analysis Using Graphics 126 (20) 6.2.2 Step 2: Model Fit Comparisons 146 (6) 6.2.3 Step 3: IE Analysis 152 (15) 6.2.3.1 All Cancer Sites Combined 153 (3) 6.2.3.2 Age Effects by Site 156 (5) 6.2.3.3 Period Effects by Site 161 (4) 6.2.3.4 Cohort Effects on Cancer Incidence 165 (1) 6.2.3.5 Cohort Effects on Cancer Mortality 166 (1) 6.2.4 Summary and Discussion of Findings 167 (2) 6.3 APC Model-Based Demographic Projection and Forecasting 169 (19) 6.3.1 Two-Dimensional versus Three-Dimensional View 170 (1) 6.3.2 Forecasting of the U.S. Cancer Mortality Trends for Leading Causes of Death 171 (37) 6.3.2.1 Methods of Extrapolation 171 (1) 6.3.2.2 Prediction Intervals 172 (1) 6.3.2.3 Internal Validation 173 (8) 6.3.2.4 Forecasting Results 181 (7) Appendix 6.1: The Bootstrap Method Using a Residual Resampling Scheme for Prediction Intervals 188 (1) References 189 (2) 7 Mixed Effects Models: Hierarchical APC-Cross-Classified Random Effects Models (HAPC-CCREM), Part I: The Basics 191 (40) 7.1 Introduction 191 (1) 7.2 Beyond the Identification Problem 192 (3) 7.3 Basic Model Specification 195 (4) 7.4 Fixed versus Random Effects HAPC Specifications 199 (6) 7.5 Interpretation of Model Estimates 205 (3) 7.6 Assessing the Significance of Random Period and Cohort Effects 208 (14) 7.6.1 HAPC Linear Mixed Models 209 (6) 7.6.1.1 Step 1: Study the Patterns and Statistical Significance of the Individual Estimated Coefficients for Time Periods and Birth Cohorts 209 (3) 7.6.1.2 Step 2: Test for the Statistical Significance of the Period and Cohort Effects Taken as a Group 212 (3) 7.6.2 HAPC Generalized Linear Mixed Models 215 (7) 7.7 Random Coefficients HAPC-CCREM 222 (5) Appendix 7.1: Matrix Algebra Representations of Linear Mixed Models and Generalized Linear Mixed Models 227 (2) References 229 (2) 8 Mixed Effects Models: Hierarchical APC-Cross-Classified Random Effects Models (HAPC-CCREM), Part II: Advanced Analyses 231 (54) 8.1 Introduction 231 (1) 8.2 Level 2 Covariates: Age and Temporal Changes in Social Inequalities in Happiness 231 (12) 8.3 HAPC-CCREM Analysis of Aggregate Rate Data on Cancer Incidence and Mortality 243 (18) 8.3.1 Trends in Age, Period, and Cohort Variations: Comparison with the IE Analysis 243 (1) 8.3.2 Sex and Race Differentials 244 (13) 8.3.3 Cohort and Period Mechanisms: Cigarette Smoking, Obesity, Hormone Replacement Therapy, and Mammography 257 (4) 8.4 Full Bayesian Estimation 261 (8) 8.4.1 REML-EB Estimation 261 (3) 8.4.2 Gibbs Sampling and MCMC Estimation 264 (4) 8.4.3 Discussion and Summary 268 (1) 8.5 HAPC-Variance Function Regression 269 (13) 8.5.1 Variance Function Regression: A Brief Overview 270 (1) 8.5.2 Research Topic: Changing Health Disparities 271 (1) 8.5.3 Intersecting the HAPC and VFR Models 272 (3) 8.5.4 Results: Variations in Health and Health Disparities by Age, Period, and Cohort, 1984-2007 275 (5) 8.5.5 Summary 280 (2) References 282 (3) 9 Mixed Effects Models: Hierarchical APC-Growth Curve Analysis of Prospective Cohort Data 285 (28) 9.1 Introduction 285 (2) 9.2 Intercohort Variations in Age Trajectories 287 (7) 9.2.1 Hypothesis 287 (1) 9.2.2 Model Specification 288 (3) 9.2.3 Results 291 (3) 9.3 Intracohort Heterogeneity in Age Trajectories 294 (6) 9.3.1 Hypothesis 294 (2) 9.3.2 Results 296 (4) 9.4 Intercohort Variations in Intracohort Heterogeneity Patterns 300 (7) 9.4.1 Hypothesis 300 (1) 9.4.2 Model Specification 301 (1) 9.4.3 Results 302 (5) 9.5 Summary 307 (2) References 309 (4) 10 Directions for Future Research and Conclusion 313 (10) 10.1 Introduction 313 (2) 10.2 Additional Models 315 (2) 10.2.1 The Smoothing Cohort Model and Nonparametric Methods 315 (1) 10.2.2 The Continuously Evolving Cohort Effects Model 316 (1) 10.3 Longitudinal Cohort Analysis of Balanced Cohort Designs of Age Trajectories 317 (2) 10.4 Conclusion 319 (1) References 320 (3) Index
Özet:
Age-Period-Cohort Analysis: New Models, Methods, and Empirical Applications is based on a decade of the authors’ collaborative work in age-period-cohort (APC) analysis. Within a single, consistent HAPC-GLMM statistical modeling framework, the authors synthesize APC models and methods for three research designs: age-by-time period tables of population rates or proportions, repeated cross-section sample surveys, and accelerated longitudinal panel studies. The authors show how the empirical application of the models to various problems leads to many fascinating findings on how outcome variables develop along the age, period, and cohort dimensions. The book makes two essential contributions to quantitative studies of time-related change. Through the introduction of the GLMM framework, it shows how innovative estimation methods and new model specifications can be used to tackle the "model identification problem" that has hampered the development and empirical application of APC analysis. The book also addresses the major criticism against APC analysis by explaining the use of new models within the GLMM framework to uncover mechanisms underlying age patterns and temporal trends. Encompassing both methodological expositions and empirical studies, this book explores the ways in which statistical models, methods, and research designs can be used to open new possibilities for APC analysis. It compares new and existing models and methods and provides useful guidelines on how to conduct APC analysis. For empirical illustrations, the text incorporates examples from a variety of disciplines, such as sociology, demography, and epidemiology. Along with details on empirical analyses, software and programs to estimate the models are available on the book’s web page.
Yazar Ek Girişi:
Copies:
Mevcut:*
Library | Materyal Türü | Demirbaş | Yer Numarası | Durumu / Lokasyon / İade Tarihi |
---|---|---|---|---|
Arıyor... | Kitap | EKOBKN0001121 | 001.422 YAN 2013 | Arıyor... |